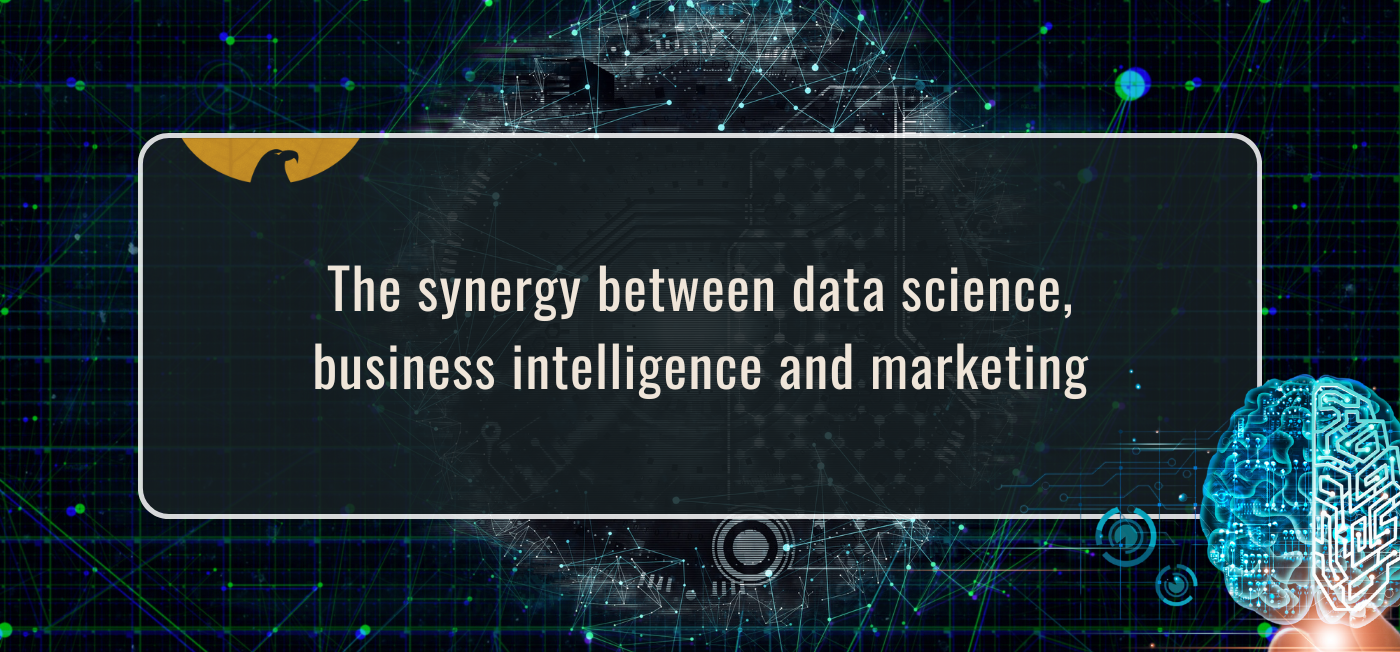
The synergy between data science, business intelligence and marketing
Did you know? According to a survey by Forbes, 53% of companies are using big data analytics today, up from 17% in 2015. In today’s dynamic business landscape, the intersection of data science, Business Intelligence (BI) and marketing has become the epicenter of power of data-driven making and competitive advantage.
A study by McKinsey and Company found that companies in the top quartile for data-driven decision-making were 5% more productive and 6% more profitable than their competitors. Harnessing the power of data-driven insights has transformed how businesses operate, optimize processes and engage with their target audience.
This month, Vantage will delve into the symbolic relationship between data science, BI and marketing, exploring how these disciplines converge to drive growth, innovation and customer-centric strategies.
Transforming business intelligence and marketing with data science
Data science, in a business context, involves extracting knowledge and insights from large datasets to solve complex problems and make data-driven decisions. The process combines various disciplines, including statistics, mathematics and computer science to analyze vast amounts of data and derive actionable insights.
On the other hand, business intelligence refers to the strategies, tools and technologies that organizations use to collect, analyze and present business information. The goal of business intelligence is to facilitate informed decision-making and improve the overall performance and efficiency of a company.
Data science and business intelligence play the same role and yet they are different. Data science is the backbone of business intelligence. Both work with data but in a very different manner.
Business Intelligence focuses on getting the historical data to understand the patterns and trends. On the other hand, data science analyzes the data to make future predictions and find new insights for achieving the goal.
If data science and Business Intelligence work together they simultaneously provide a perspective and predicate the insights of the business and can enhance the level of marketing.
Role of Data Science in Business Intelligence
Data Science plays a pivotal role in Business Intelligence (BI) by leveraging advanced analytics techniques to extract meaningful insights from data and support decision-making processes.
Customer segmentation
Data Science techniques like clustering algorithms can be used to segment customers based on their behavior, preferences and buying patterns. These segments can then be utilized in BI dashboards to tailor marketing strategies, promotions and product offerings.
For example – A retail company may use customer segmentation to create targeted campaigns for different customer groups, leading to improved sales and customer satisfaction.
Predictive maintenance
Data Science models can analyze sensor data from machinery and equipment to predict maintenance needs and prevent costly downtime. These predictive maintenance insights can be integrated into BI systems to provide real-time monitoring of equipment health, schedule proactive maintenance and optimize asset performance.
Manufacturing companies can use predictive maintenance in Business Intelligence (BI) dashboards to reduce equipment failure, increase operational efficiency and lower maintenance costs.
Fraud detection
Data Science algorithms can detect automobiles and patterns indicative of fraudulent activities in financial transactions, insurance claims or online transactions. By integrating fraud detection models into Business Intelligence (BI) systems, organizations can monitor and analyze large volumes of transactional data in real-time, flag suspicious activities and take immediate action.
Banking sector can use BI dashboards with Data Science-powered fraud detection to identify and prevent fraudulent transactions, enhancing security and trust among customers.
Demand forecasting
Data Science techniques like time series forecasting can predict future demand for products or services based on historical sales data, market trends and external factors. These demand forecasting models can be integrated into BI platforms to provide accurate sales forecasts, optimize inventory management and support strategic decision-making.
E-commerce companies can leverage BI dashboards with demand forecasting capabilities to ensure sufficient stock levels, reduce stockouts and improve customer satisfaction.
Role of Data Science in Marketing
Data Science plays a crucial role in modern marketing strategies by leveraging data analytics, machine learning and statistical modeling to drive data-driven decision-making, optimize campaigns and enhance customer experiences.
Customer segmentation and targeting
Data Science enables marketers to segment customers based on demographics, behavior, preferences and purchase history. This segmentation helps create targeted marketing campaigns, promotions and product recommendations.
Predictive analytics for customer behavior
Data Science models predict customer behavior, such as purchase likelihood, churn risk and lifetime value. This enables marketers to tailor strategies and retain valuable customers and optimize marketing spend.
OTT platforms like Netflix predict viewer preferences to recommend relevant content and reduce subscriber churn.
Companies like Amazon and Flipkart segment customers based on their browsing and purchase history to recommend personalized products and promotions.
Recommendation systems
Recommendation engines powered by Data Science analyze user interactions to suggest personalized products, services or content.
For example
Music applications like Spotify recommend music playlists based on a user’s listening habits and YouTube suggests videos based on viewing history and preferences.
Campaign sentiment analysis
Sentiment analysis tools powered by Data Science analyze customer feedback from social media, reviews and surveys to gauge sentiment and identify trends. Marketers use this data to understand customer perceptions, manage brand reputation and tailor messaging accordingly.
An example is X sentiment analysis used by brands to monitor brand mentions and sentiment in real-time.
Marketing attribution
Data Science helps attribute conversions and sales to specific marketing touchpoints, channels or campaigns. Marketers use attribution models to measure the impact of each marketing effort and optimize budget allocation.
Google Analytics offers attribution modeling to track customer journeys and measure the contribution of different channels to conversions.
The power of collaboration – data science business intelligence
While Data Science and Business Intelligence (BI) have distinct methodologies, their collaboration is key to unlocking comprehensive business insights and driving marketing success.
Scope and focus
Data science and business intelligence work together to predict after analyzing the data. Business Intelligence (BI) has some typical methods and insights. It deals with structured data and keeps the focus on generating reports, dashboards and visualizations. If I talk about data science, it mainly focuses on encompassing a broader range of techniques and methodologies. It includes advanced statistical analysis, machine learning and enhanced predicting tools, to understand the deep insights of the market.
The complexity of data
If the data is increasing and becoming complicated it becomes sometimes difficult to extract meaningful data from the business. Data science helps the business by extracting important information and data from complex and unstructured data. Sometimes a sophisticated algorithm is important to get used to get the data and information. While BI has expertise in working with structured data and predefined frameworks.
Predictive analytics and machine learning
To understand the data, patterns and trends predictive analytics is important to work. Predictive analytics and machine learning are the key features where Business Intelligence and Data Science is designed to build models that are predictive and used for long-term prediction, identify patterns and make data-driven predictions.
These capabilities give value to the work and make the business give the competition. Whereas BI professionals work on getting historical data with the help of established methodologies and reporting frameworks.
Innovation and agility
Data scientists always explore new technologies and work on new algorithms, extracting new patterns and trends. They use the tools to predict the data, extract insights from the data and create innovations within their organizations. While BI professionals mostly focus on working with established methodologies, and reporting frameworks, it may not always be sufficient if they want to follow modern business trends.
Business Value Creation
Ultimately the goal of BI professionals and Data scientists is to increase the business value but their method of doing this is different. Data scientists try to unlock new features and ideas to find more ideas and innovative ways to enhance the value of the business.
They leverage predictive modeling, and innovative solutions to transform complex businesses into simple yet so attractive ones. Whereas BI professionals focus on establishing actionable insights for operational and strategic decision-making, but within predefined frameworks and reporting structures.
In conclusion
In the realm of modern business, Data Science, Business Intelligence and Marketing form a symbiotic relationship driving strategic decision-making, customer engagement and business growth. By harnessing the strengths of each discipline and fostering collaboration, organizations can unlock actionable insights, innovate their strategies and stay competitive in today’s data-driven landscape.
Overall data science is playing the perfect role in business intelligence and marketing and it will be a great opportunity for marketers to use data science and business intelligence together in the market to get a good number of customers in the market. Their work process is different but simultaneously they can be the best match. Established methods and modern techniques help know the patterns of the trends, markets, etc, and can find a good way to implement them into the market.